Innovative Advances in Content Similarity Detection Technology
Written on
Chapter 1: The Importance of Content Similarity Detection
In today's digital landscape, where an overwhelming amount of information is available, the detection of content similarity has become crucial. This capability is vital for addressing plagiarism in educational settings and ensuring creativity in various industries. Recent strides in this area have been transformative, driven by groundbreaking research and innovative technologies.
Researchers are actively investigating deep learning methodologies, including neural networks and natural language processing (NLP) algorithms, to improve content similarity detection. By utilizing expansive datasets, these systems can uncover complex patterns within text, leading to enhanced accuracy in results. Furthermore, advancements in machine learning models, such as BERT (Bidirectional Encoder Representations from Transformers), have considerably advanced contextual comprehension, enabling the detection of subtle similarities that were once difficult to uncover.
This paragraph will result in an indented block of text, typically used for quoting other text.
Section 1.1: Breakthroughs in Unsupervised Learning
A significant breakthrough has emerged in unsupervised learning, allowing algorithms to identify similarities without the need for labeled datasets. This approach not only streamlines the process but also facilitates the detection of similarities in previously uncharted areas.
Subsection 1.1.1: The Role of Semantic Analysis
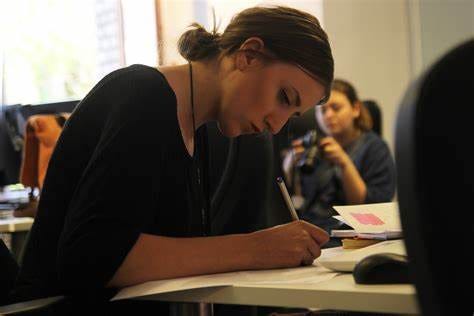
The incorporation of semantic analysis has further strengthened content similarity detection. By understanding the meanings of words and phrases, algorithms can better ascertain content-relatedness. This capability is particularly crucial in sectors such as healthcare, legal affairs, and finance, where precise interpretation is vital.
Section 1.2: Addressing Paraphrasing Challenges
In the academic realm, recent studies focus on developing algorithms that can detect paraphrased content—a prevalent issue in plagiarism assessment. By examining both syntactic and semantic structures, these algorithms can identify subtle rewording, ensuring a more thorough and precise evaluation of content similarity.
Chapter 2: Innovations in Content Verification
The first video titled "Can Turnitin detect AI research work || AI feature Plagiarism checker" delves into the capabilities of AI tools in recognizing and addressing plagiarism in academic work. This video provides insights into how these technologies are evolving to meet contemporary challenges.
The second video, "20 best plagiarism checker and AI Content detector," reviews various tools available for detecting plagiarism and AI-generated content. It highlights the best options for individuals and institutions seeking to uphold originality in their work.
Moreover, the emergence of blockchain technology has introduced innovative techniques for verifying the authenticity of digital content. By establishing unalterable records of content ownership and modifications, blockchain technology not only deters plagiarism but also provides a clear and secure framework for content creators and users.
In summary, the field of content similarity detection is swiftly advancing, propelled by exceptional research and technological advancements. These innovations not only empower educators, creators, and professionals but also foster a more genuine and original digital environment. As we progress, it is essential to stay informed about these developments, ensuring our ability to navigate the intricate web of digital information with confidence and integrity.
If you found this information useful, please consider giving me claps and following for more updates. Your support is greatly appreciated.