Innovative AI: Paving the Way for Future Scientific Discoveries
Written on
Chapter 1: The Intersection of AI and Scientific Discovery
The vision of limitless experimentation fueling scientific breakthroughs has long captivated the human imagination, tracing back to the eerie tales of Frankenstein. This fascination has evolved into today's intrigue with artificial intelligence (AI), which seeks to create an animated intellect devoid of a physical form. But can AI genuinely streamline the process of unearthing new scientific knowledge? A recent investigation assessed the potential of contemporary large language models (LLMs) in enhancing scientific research.
The challenge of modern scientific inquiry is substantial, as the volume of new research publications surges each year. The sheer volume of information generated makes it impossible for any researcher to keep pace. Beyond our cognitive limitations lies an expansive realm of untapped ideas and innovations. While humans excel at synthesizing diverse viewpoints, our ability to process this burgeoning knowledge is constrained.
Tom Hope, a researcher at the Allen Institute for Artificial Intelligence and co-author of the recent study "Scientific Inspiration Machines Optimized for Novelty" (SCIMON), recognized the need to tackle this intriguing challenge. SCIMON is a pioneering framework designed to leverage the power of LLMs for scientific exploration, aiming to enhance human creativity by sourcing and synthesizing novel scientific concepts from extensive databases of existing research.
“Our cognitive abilities, while incredibly powerful, still struggle to navigate the vast landscape of ideas and possibilities.” — Tom Hope
Section 1.1: Introducing SCIMON
Enter SCIMON, developed to elevate the scientific discovery process. This framework harnesses LLMs to tap into the collective intelligence embedded in scientific literature, assisting researchers in uncovering transformative insights and proposing innovative research paths. This state-of-the-art tool marks a significant advancement in employing AI to expedite and enrich scientific inquiry.
In contrast to traditional AI models that focus solely on binary link predictions, SCIMON adopts a more comprehensive approach by integrating problem contexts and iteratively generating inventive ideas.
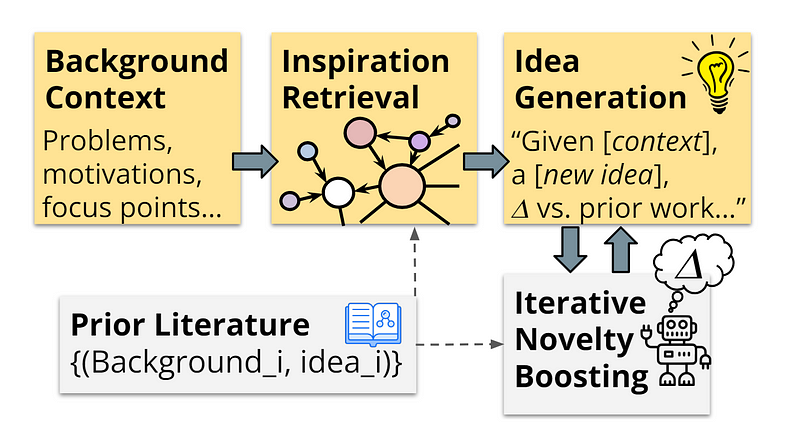
SCIMON functions through a complex framework that involves several essential components. Initially, it inputs detailed background information, encompassing current challenges, experimental contexts, and research motivations. This context is crucial for SCIMON’s inspiration retrieval process, which examines past scientific literature for relevant ideas and solutions.
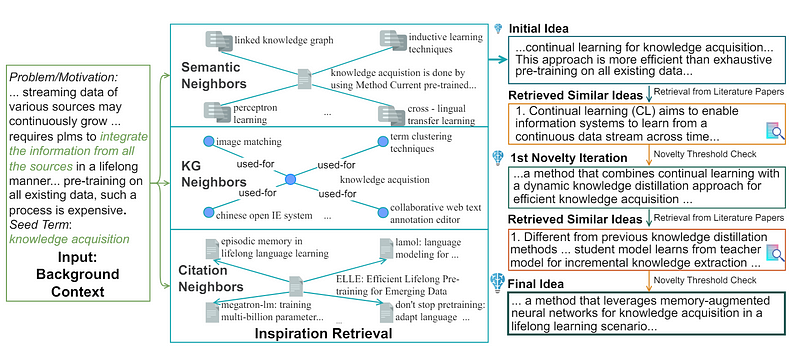
“By framing our problems in a way that allows us to extract valuable knowledge from extensive literature, we can synthesize actionable insights that support decision-making and potentially automate some of these processes.” — Tom Hope
The inspirations retrieved by SCIMON are not merely repeated; they act as seeds for generating new concepts. The system iteratively compares these generated ideas against existing research, optimizing for novelty at each stage. This process ensures that the final output is both relevant and original, pushing the boundaries of current scientific knowledge.
For instance, the SCIMON team illustrated a scenario where the system addressed a problem related to optimizing complex functions. It drew inspiration from thermodynamics and swarm intelligence, leading to innovative hypotheses about optimization techniques grounded in natural analogies.
Section 1.2: Experimental Insights
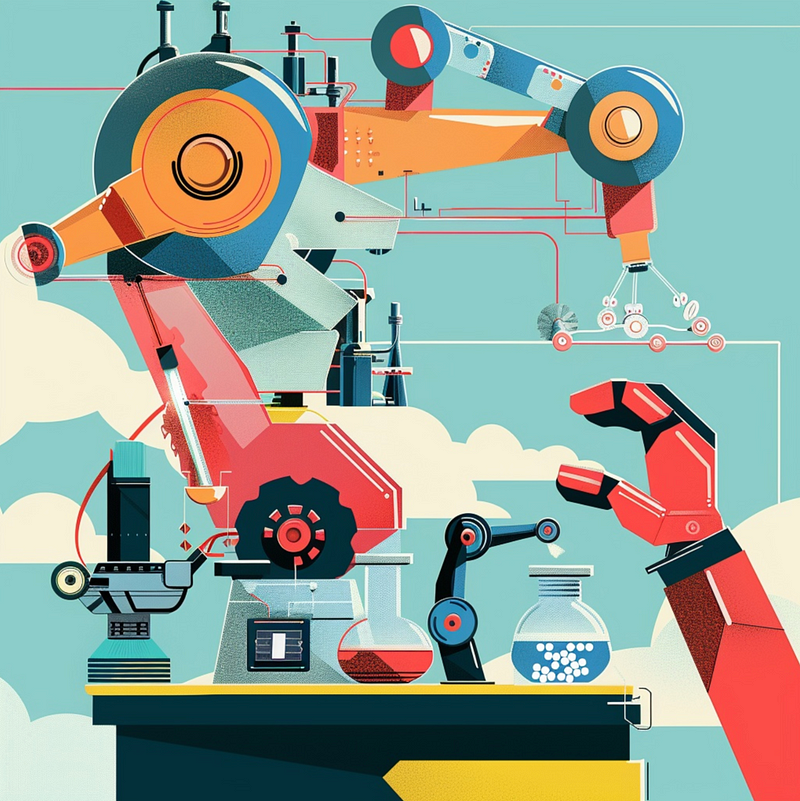
In extensive evaluations, SCIMON was compared to leading LLMs, such as GPT-4. The results were encouraging — while standard LLMs frequently generated generic or incremental ideas, SCIMON's methodologies significantly enhanced the novelty and relevance of the proposed hypotheses. The iterative novelty-boosting mechanism played a critical role in refining ideas to ensure they were genuinely innovative.
Several successful case studies detailed in the paper illustrate SCIMON’s potential to facilitate groundbreaking scientific discoveries:
General NLP Domain Experiment
In one experiment, SCIMON was assigned the task of proposing novel research directions in natural language processing. It retrieved related studies on dynamic knowledge distillation and suggested an approach that combines lifelong learning with dynamic adaptation techniques, a novel concept recognized as feasible and inventive by human experts.
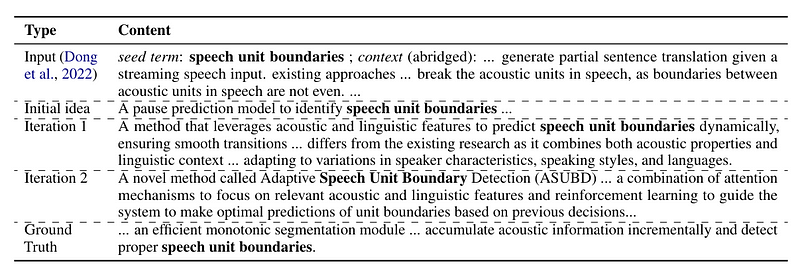
Model Variants: Various configurations were tested, including GPT3.5FS, GPT4FS, and T5+SN+CL.
Outcome: The GPT4FS and GPT4FS+KG models significantly outperformed others in terms of novelty and technical depth, generating ideas that were considerably distinct from the original context and receiving high ratings from human evaluators.
Biomedical Domain Case Study
In the biomedical arena, the Meditron-7b model was fine-tuned on a PubMed dataset and evaluated on a post-training set. Human evaluators rated 80% of the AI-generated research directions favorably, often finding the AI's suggestions to be more detailed and technically advanced than the initial concepts.
Model Used: Meditron-7b.
Procedure: The model was fine-tuned on a large dataset sourced from PubMed and assessed on a test set beyond its pre-training cutoff date.
Outcome: Human evaluators with graduate-level education in biochemistry rated 80% of the proposed directions positively, highlighting a greater satisfaction with the AI-generated outputs compared to traditional research ideas.
Iterative Novelty Boosting with Retrieval
SCIMON utilized an iterative mechanism to enhance the novelty of research ideas. For example, an initial suggestion for predicting speech unit boundaries evolved into a proposal for Adaptive Speech Unit Boundary Detection (ASUBD), incorporating attention mechanisms and reinforcement learning, which experts deemed highly innovative.
Evaluation of Iterative Novelty Boosting
SCIMON’s iterative novelty-boosting technique was assessed for its effectiveness in enhancing research idea novelty. Human annotators indicated that the revised ideas were often more creative and original than the initial suggestions. This iterative approach enabled SCIMON to refine its outputs, making them more distinct and innovative, which was well-received by field experts.
Chapter 2: The Promising Future of SCIMON
The potential implications of SCIMON's capabilities are significant. By automating elements of hypothesis generation, SCIMON can assist researchers in navigating the overwhelming scientific literature, revealing hidden connections, and proposing fresh research avenues. This not only accelerates the pace of discovery but also democratizes access to leading-edge scientific insights.
“LLMs can act as extensions of our capabilities, enhancing how we search for relevant knowledge and inspiration, allowing us to discover insights we might otherwise overlook.” — Tom Hope.
While the aspiration for fully automated scientific discovery remains a future goal, SCIMON represents a considerable stride toward achieving this vision. The synergy between AI and human researchers opens new frontiers in science, where AI can amplify human creativity and decision-making.
SCIMON illustrates that with appropriate frameworks and iterative processes, AI can assist in generating new scientific knowledge, pushing the limits of our understanding and accelerating the journey toward future breakthroughs.
The first video, "Future of Science and Technology Q&A (January 5, 2024)," discusses the transformative potential of AI in scientific research and addresses viewer questions about its implications.
The second video, "Session 3 - Scientific Advances powered by AI," highlights the latest advancements in science facilitated by AI technologies, showcasing real-world applications and future possibilities.

This article is published on Generative AI. Connect with us on LinkedIn and follow Zeniteq to stay updated with the latest AI narratives. Subscribe to our newsletter for ongoing updates in the realm of generative AI. Together, let’s shape the future of AI!
